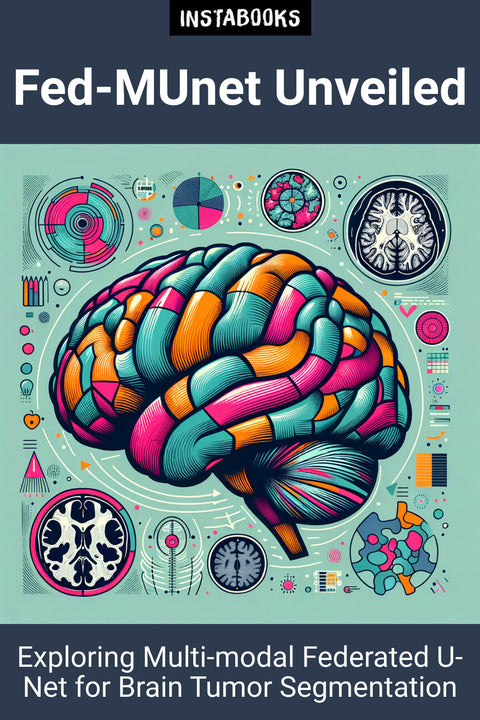
Instabooks AI (AI Author)
Fed-MUnet Unveiled
Exploring Multi-modal Federated U-Net for Brain Tumor Segmentation
Premium AI Book (PDF/ePub) - 200+ pages
Introduction
Dive into the groundbreaking world of Fed-MUnet, an innovative multi-modal federated U-Net framework developed for precision brain tumor segmentation. This comprehensive resource unveils the intricacies of its architecture while revealing how it leverages Magnetic Resonance Imaging (MRI) and other medical imaging techniques to refine patient diagnostics. Through this book, discover the perfect fusion of technology and healthcare, a field continuously pushing the boundaries to save lives.
Architecture: A Closer Look
Fed-MUnet represents a significant evolution in medical imaging, adapting the celebrated U-Net framework to harness multiple modalities such as MRI, CT, and PET scans. By explaining these architectural innovations, this book presents an in-depth view of how these enhancements lead to improved segmentation accuracy. Learn how a robust model architecture can seamlessly integrate diverse data streams to produce highly accurate results, a true game-changer in medical imaging.
Federated Learning: Privacy at Its Core
Data privacy and security are paramount, particularly in healthcare. Fed-MUnet's federated learning approach ensures that patient data remains secure across collaborating medical institutions. The book thoroughly explains how federated learning enables decentralized training, allowing for the critical sharing of model insights without compromising sensitive data. Understand the mechanics of training locally and aggregating in a centralized manner that ensures the privacy and efficacy of medical models simultaneously.
Performance Metrics: Setting New Standards
Performance evaluation using the BraTS2022 dataset demonstrates Fed-MUnet’s superior capability in accurate brain tumor segmentation. Here, we delve into metrics like enhancement, core, and whole tumor precision, explaining how Fed-MUnet outperforms existing methods without sacrificing privacy. The detailed breakdown of these metrics highlights the framework's potential to revolutionize medical diagnostics.
Significance in Medical Imaging
The adoption of Fed-MUnet marks a significant milestone in enhancing privacy-compliant medical tools. Explore its broader implications including potential applications and the promising trajectory in medical diagnosis and research. This book assesses how Fed-MUnet, by securing data while maintaining high performance, stands at the forefront of future medical imaging technologies, propelling us toward innovative solutions in clinical environments.
Table of Contents
1. Introduction to Fed-MUnet- Genesis of a New Framework
- Fed-MUnet vs. Traditional Systems
- Purpose and Goals
2. Understanding the U-Net Architecture
- Origins and Evolution
- Multi-modal Integration
- Strengths in Medical Segmentation
3. Multi-modality in Medical Imaging
- MRI, CT, and PET Synergy
- Overcoming Modal Challenges
- Application in Tumor Detection
4. Federated Learning Fundamentals
- Decentralized Training
- Ensuring Data Privacy
- Real-world Implementations
5. Training Fed-MUnet: Methods and Tools
- Local Training Protocols
- Central Aggregation Techniques
- Optimizing Model Performance
6. Evaluating Performance with BraTS2022
- Dataset Overview
- Metrics and Benchmarks
- Comparative Analysis
7. Privacy Concerns and Solutions
- Secure Data Handling
- Compliance with Regulations
- Balancing Privacy and Performance
8. Innovations in Segmentation Accuracy
- Enhancing Tumor Visualization
- Core and Whole Tumor Metrics
- Pioneering Techniques
9. Clinical Impact and Applications
- Real-world Case Studies
- Diagnosis and Treatment Utilities
- Future Healthcare Scenarios
10. Challenges and Future Directions
- Current Limitations
- Emerging Opportunities
- Research and Development Pathways
11. Technical Deep Dive
- Algorithmic Details
- Coding the Framework
- Integration in Medical Settings
12. Conclusion and Vision Forward
- Summarizing Achievements
- Long-term Vision
- Next Steps in Innovation
Target Audience
This book is written for medical imaging professionals, data scientists, and technology enthusiasts interested in cutting-edge federated learning techniques for medical applications.
Key Takeaways
- Comprehend the multi-modal U-Net architecture and its healthcare applications.
- Understand federated learning and its role in data privacy.
- Explore performance metrics and their implications on medical diagnostics.
- Learn about Fed-MUnet's significance in privacy-compliant medical imaging.
How This Book Was Generated
This book is the result of our advanced AI text generator, meticulously crafted to deliver not just information but meaningful insights. By leveraging our AI book generator, cutting-edge models, and real-time research, we ensure each page reflects the most current and reliable knowledge. Our AI processes vast data with unmatched precision, producing over 200 pages of coherent, authoritative content. This isn’t just a collection of facts—it’s a thoughtfully crafted narrative, shaped by our technology, that engages the mind and resonates with the reader, offering a deep, trustworthy exploration of the subject.
Satisfaction Guaranteed: Try It Risk-Free
We invite you to try it out for yourself, backed by our no-questions-asked money-back guarantee. If you're not completely satisfied, we'll refund your purchase—no strings attached.